Introduction
Amazon Rufus is transforming the way shoppers navigate Amazon’s vast catalog by offering real-time, AI-driven product recommendations tailored to specific needs. In our previous blog article, Testing Amazon Rufus: Insights and Tips to Maximize Product Visibility, we explored Rufus’s functionality and overall capabilities.
Building on these insights, we conducted a larger statistical analysis of Rufus’s recommendations, uncovering patterns in the data to better understand what factors influence its selections. By analyzing key listing attributes such as content, ratings, and fulfillment methods, we aim to provide insights into how sellers and vendors can optimize their listings to align with Rufus’s advanced recommendation system. If you haven’t yet, check out our first article for foundational insights before diving into this data-driven exploration.
Our Analysis Approach
To uncover patterns in Rufus’s recommendations, we designed a methodology that involved analyzing more than 1,300 product listings. Here’s how we approached it:
Step 1: Collecting Search Terms
We sourced the top 500 generic search terms from Amazon’s Search Term Report for the U.S. marketplace. These terms reflect high-volume queries spanning a wide range of categories, from seasonal items to everyday essentials. Examples include:
- Christmas decorations indoor
- Chapstick
- Phone holders for your car
“Generic” means that the search terms do not include any brand, e.g. we excluded search terms like “ps5” or “iphone 16” where the results are not expected to be very diverse.
Step 2: Generating Realistic Customer Queries
Using ChatGPT, we then crafted natural, conversational queries for each search term to simulate typical customer interactions with Rufus. Each query aimed to elicit detailed, contextually relevant recommendations. Here are a few examples:
- For the search term “Christmas decorations indoor”, the query was:
"Please recommend indoor Christmas decorations for a festive home." - For “Chapstick”, the query was:
"Please recommend a hydrating chapstick for daily use." - For “Phone holders for your car”, the query was:
"Please recommend a reliable phone holder for the car."
These queries were submitted to Rufus on the US marketplace, and its product recommendations for each term were logged.
Step 3: Logging ASIN Recommendations from Rufus
While the number of product recommendations per search query varied, we were able to collect a dataset of over 1,300 recommended ASINs for further analysis.
Step 4: Retrieving ASIN Data Using AMALYTIX
We imported the collected ASINs into our tool AMALYTIX, which provided detailed insights into each product listing’s attributes. Key metrics included:
- Content and Presentation: Metrics such as title length, bullet point usage, image count, and the presence of enhanced content (e.g., A+ or A+ Premium) were analyzed to assess the quality of the listing’s presentation and its ability to engage customers.
- Customer Feedback and Ratings: The average star rating, the total number of customer reviews, and customer sentiment trends provided insights into buyer satisfaction and trust.
- Performance and Visibility: Data on organic search rank, Prime eligibility, and fulfillment type highlighted each listing’s discoverability and competitiveness on Amazon.
Step 5: Analyzing Patterns in Product Recommendations
We examined the data to identify recurring themes and attributes among the recommended products. This analysis revealed key factors that influence Rufus’s recommendations, offering insights for sellers and vendors.
Step 6: Testing Consistency of Recommendations
To assess the consistency of Rufus’s recommendations, we selected 10 random keywords and their corresponding customer queries. Each query was run through Rufus five additional times, and the results were compared to evaluate how stable the recommendations were across repeated tests. This step provided insights into the reproducibility of Rufus’s suggestions.
Why Correlation doesn’t equal Causation
While the findings from our analysis offer valuable insights, it’s important to recognize the limitations of this approach. The patterns we observed do not necessarily indicate a direct causal relationship between specific product attributes and Rufus’s recommendations. These trends may instead reflect broader customer preferences or the way product listings are generally optimized for Amazon.
Key Patterns in Amazon Rufus Recommendations
Before we start here is a quick explanation of the key metrics we used:
- Median: The median represents the middle value in a sorted dataset, splitting it into two equal halves.
- Interquartile Range (IQR): The IQR measures the spread of the middle 50% of a dataset, calculated as the difference between the 75th percentile (Q3) and the 25th percentile (Q1). It helps identify the concentration of values and detect potential outliers.
1. Title Length
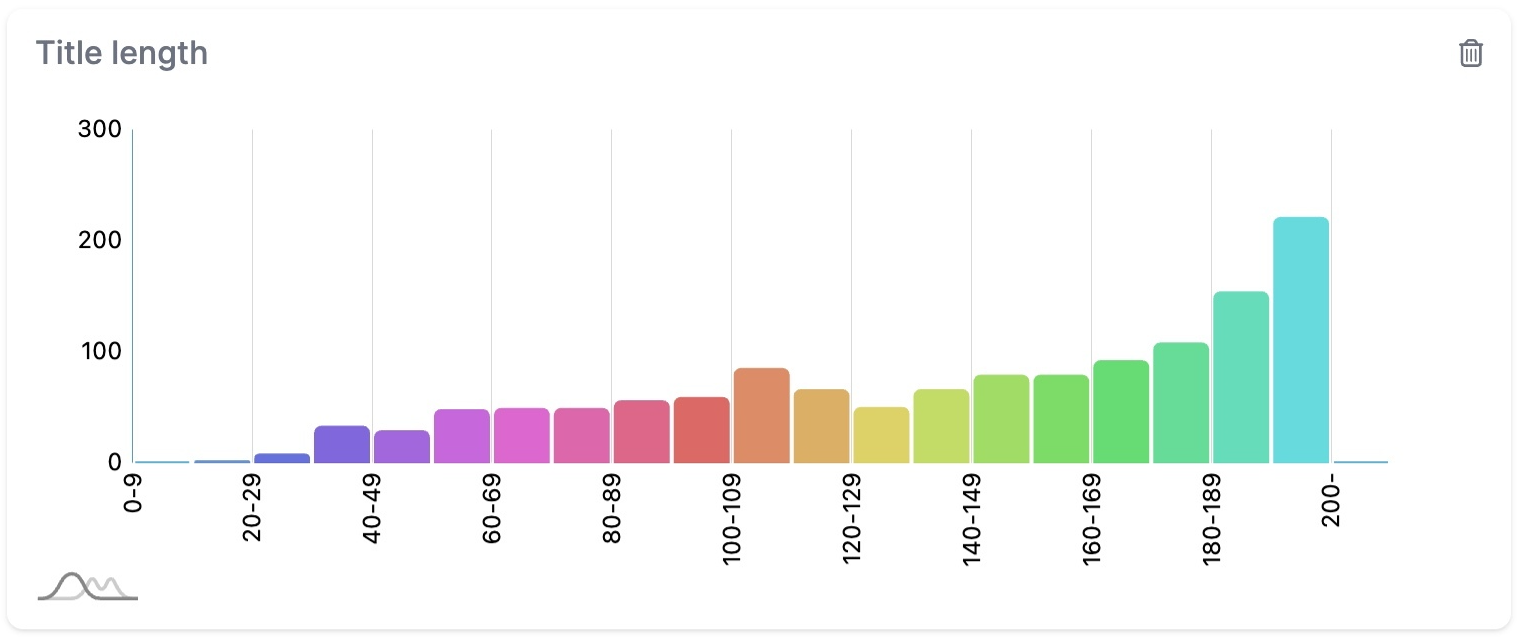
- Median Length: 166 characters
- Interquartile Range: 100 to 184 characters
While the median and mean title lengths are influenced by listings with significantly fewer characters, the majority of product titles were concentrated at the higher end of the spectrum, particularly between 190 and 199 characters. For example, 199 characters was the most common title length. This suggests that many recommended listings utilize the maximum allowable space to include detailed product information.
2. Brand Representation

The analysis revealed that 74.7% of recommended products came from distinct brands, indicating a significant level of brand diversity in Rufus’s recommendations. This suggests that Rufus does not overly favor a small number of brands and instead includes a wide range of options in its recommendations.
Amazon Basics accounted for only 0.6% of all recommended products, making it the 5th most recommended brand overall. Despite being a prominent Amazon private label, its relatively low share of recommendations suggests that Amazon does not appear to give undue preference to its own products in Rufus’s recommendations.
3. Image Count
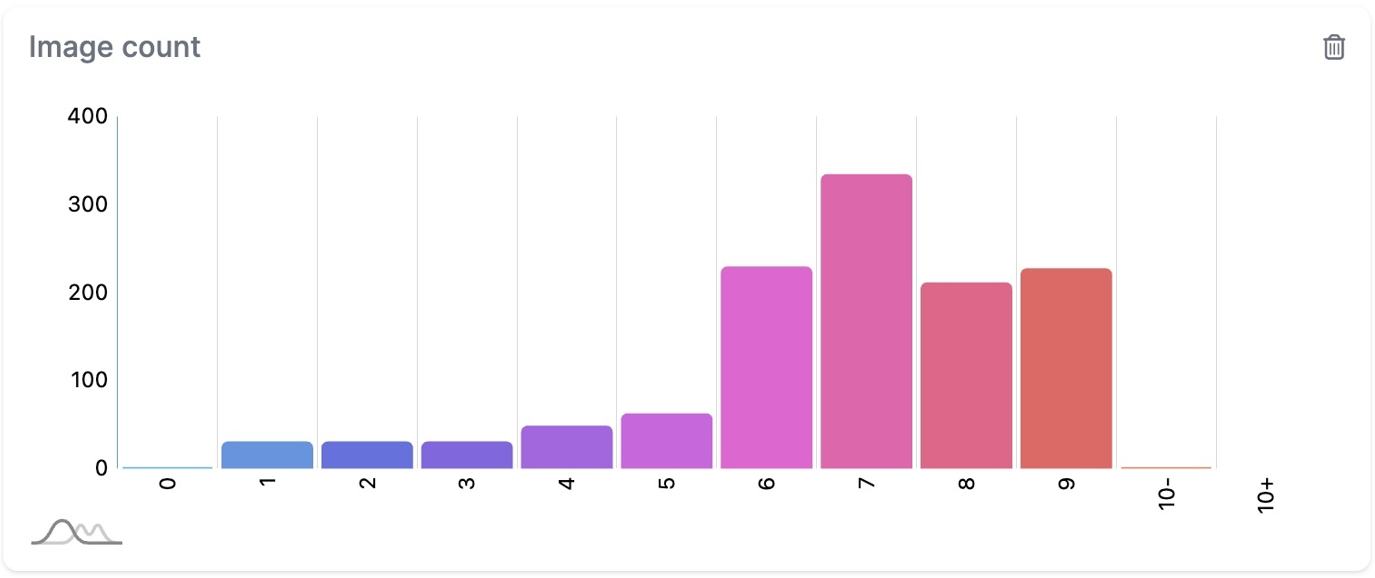
- Median Image Count: 7
- Interquartile Range: 6 to 9
The majority of products featured between 6 and 9 images, with 7 images being the most frequent configuration, present in over 25% of listings. While a small number of products included more than 10 images, these were exceptions rather than the norm. Very few listings had fewer than 4 images, indicating the importance of utilizing multiple visuals to showcase products effectively.
These findings highlight the significance of a robust visual presentation, as listings with a well-curated set of images appear prominently in Rufus’s recommendations. Brands should aim for 6–9 high-quality images to align with common trends among recommended products.
4. Video Count
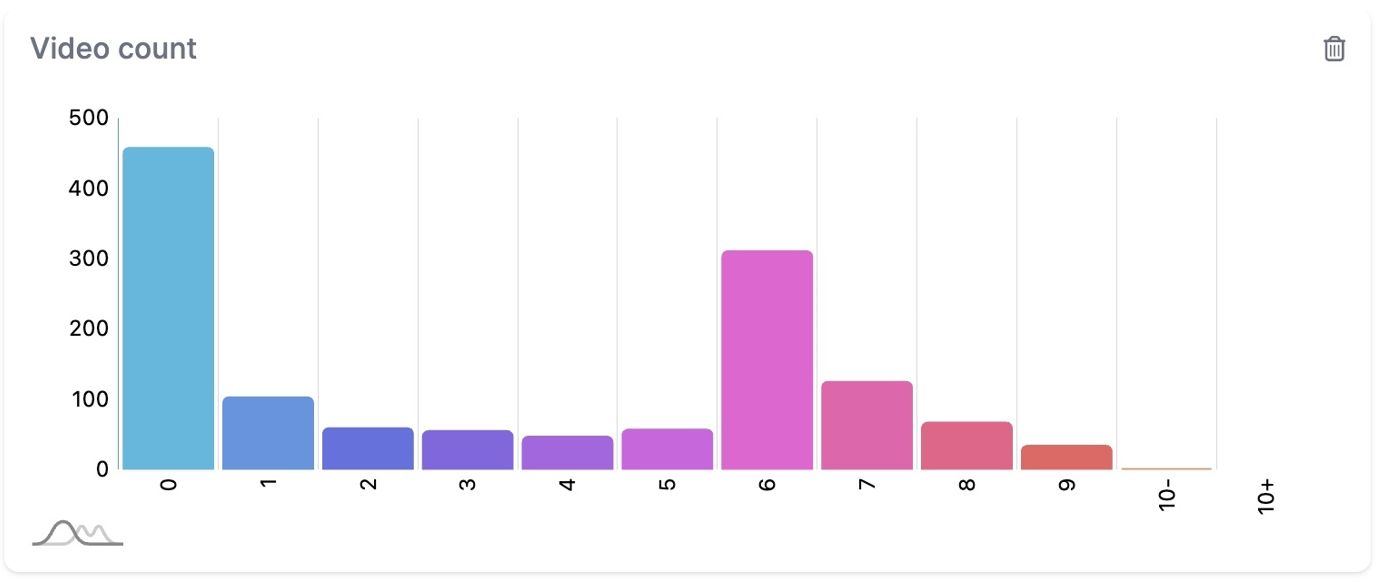
- Median Video Count: 3
- Interquartile Range: 0 to 6
While videos were present in the majority of recommended products, over 34% of listings included no videos at all. Among all recommendations, the median count was 3, and 22.9% of listings featured 6 videos, making it the most frequent non-zero value. Only a small fraction of products used the maximum of 10 videos.
These findings suggest that while having videos may enhance a product's appeal and likelihood of recommendation, their absence does not necessarily exclude a product from being recommended. However, listings with multiple videos likely gain an advantage in showcasing product details and engaging customers effectively.
5. Badges
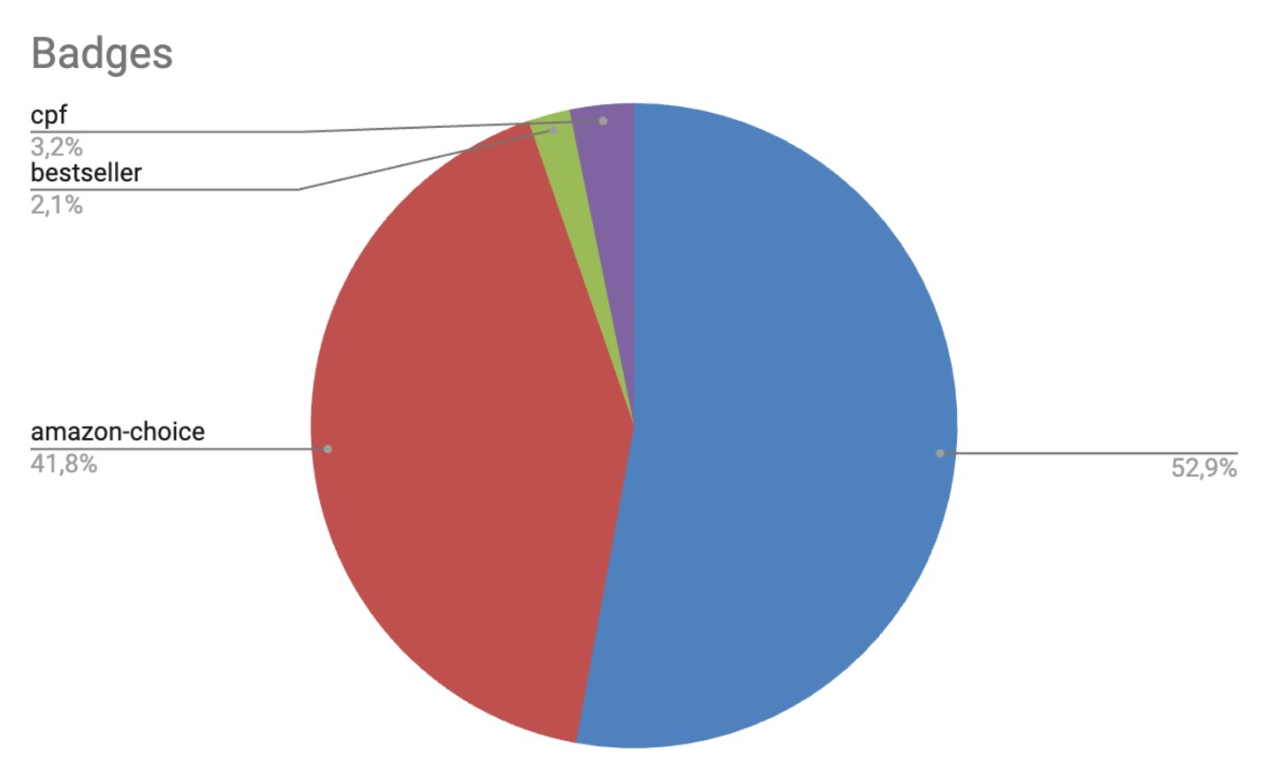
- Products With Amazon’s Choice Badge: 41.8%
- Products with CPF Label: 3.2%
A significant proportion of recommended products (41.8%) featured the Amazon’s Choice badge, indicating that this designation may play a notable role in product visibility within Rufus’s recommendations. However, the majority of recommended products (58.2%) did not have the badge, showing that while beneficial, it is not a requirement for inclusion.
For brands, obtaining the Amazon’s Choice badge could enhance a product’s chances of being recommended, especially in competitive categories.
The majority of recommended products did not carry the Climate Pledge Friendly label (CPF), with only a small proportion (3.2%) featuring this sustainability certification. This indicates that while the label may enhance a product’s appeal to eco-conscious shoppers, it is not a predominant factor in determining recommendations by Rufus.
For brands aiming to target environmentally conscious consumers, obtaining the CPF label could still serve as a valuable differentiator in specific categories.
6. Number of Bullet Points
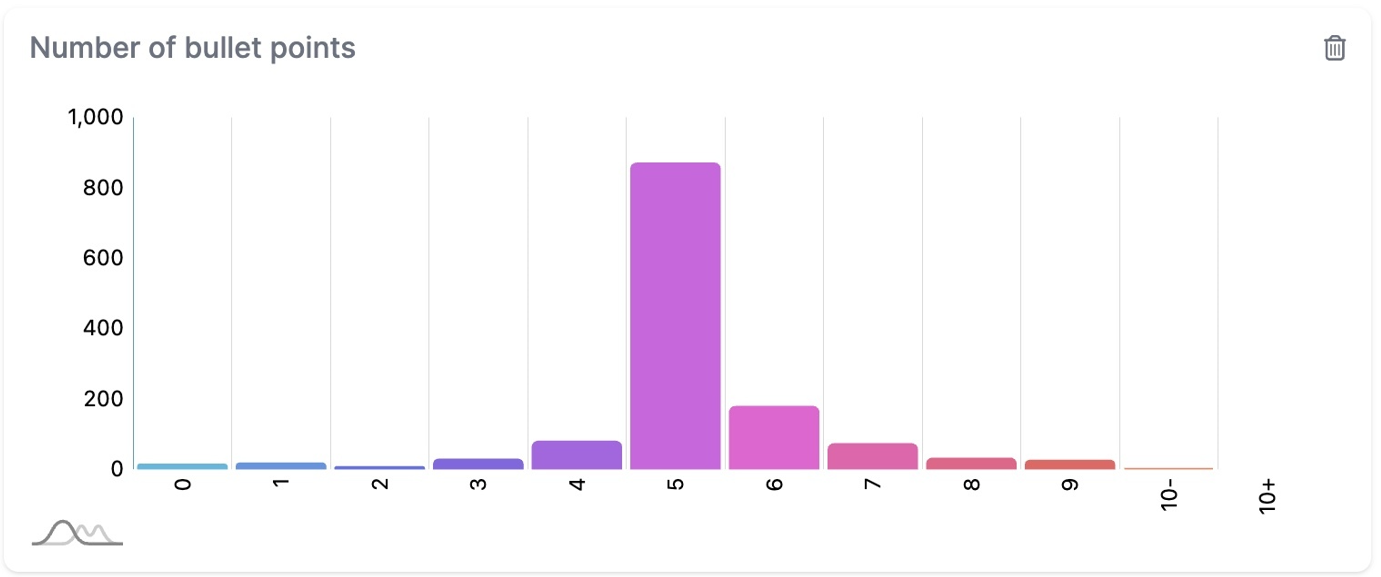
- Median Number of Bullet Points: 5
- Interquartile Range: 5 to 5
The majority of products had 5 bullet points, as reflected by the narrow interquartile range and the median aligning with this value. While some listings included up to 12 bullet points, these were less common, and only 1% of products lacked bullet points entirely.
The prevalence of listings with exactly five bullet points suggests a strong alignment with Amazon's standard practice of using five key bullet points to describe product features. This highlights the importance of utilizing bullet points effectively to convey essential product information concisely.
7. Description Type / A+ Content

- A+ Content: 55.1%
- A+ Premium Content: 32.1%
- Standard Description: 12.8%
Over half of the recommended products utilized A+ Content, while an additional 32.1% featured A+ Premium Content, leaving only 12.8% with standard descriptions. This highlights the importance of enhanced content in product listings, as the majority of recommendations leveraged visually rich and informative descriptions to engage customers.
Brands aiming to improve their product visibility in Rufus’s recommendations should consider upgrading to A+ Content or A+ Premium Content to better communicate product value and enhance the overall shopping experience.
8. Product Ratings
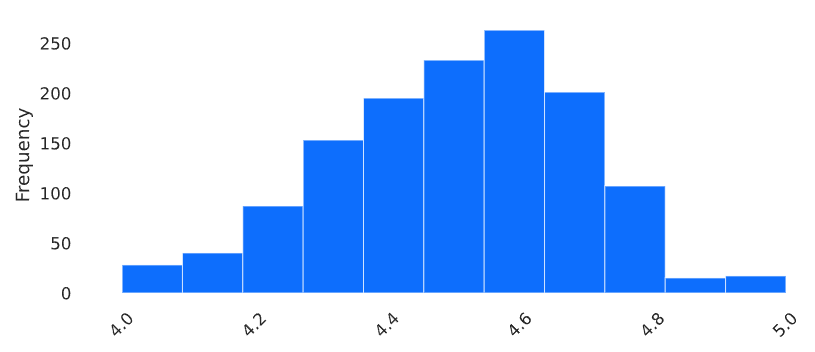
- Median Rating: 4.5
- Interquartile Range: 4.3 to 4.7
One notable finding is that Rufus does not appear to recommend products with an average rating below 4.0. The majority of recommended products had ratings of 4.3 or higher, with most concentrated around a median rating of 4.5. This indicates that high customer ratings are a prominent feature of listings recommended by Rufus, emphasizing the importance of maintaining strong product reviews and feedback.
9. Number of Reviews
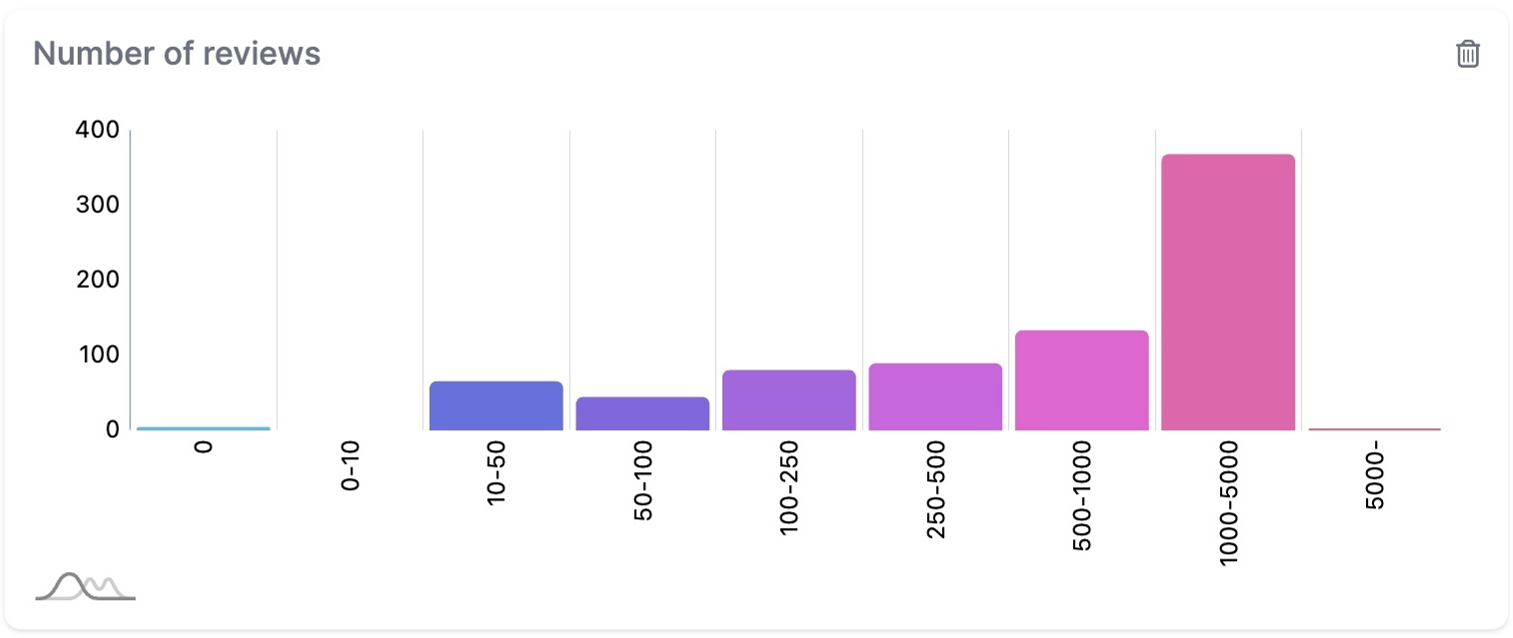
- Median Number of Reviews: 2,991
- Interquartile Range: 640 to 12,733
A significant portion of recommended products had thousands of customer reviews, with the median at nearly 3,000. Products in the upper quartile exceeded 12,732 reviews, while a small number had review counts exceeding 100,000, indicating strong customer engagement.
Interestingly, 0.1% of products had zero reviews, showing that while reviews are a critical factor, Rufus occasionally recommends products with little to no customer feedback. However, the high average and upper quartile values suggest that a substantial number of reviews may contribute to increased visibility in recommendations.
10. Organic Rank and Visibility in Amazon Search
- Median Organic Rank: 41
A significant portion of recommended products had strong organic visibility in the traditional Amazon search for their respective keyword, with ranks clustered below the median of 41. Products with organic ranks in the top 10 were prevalent, with 7.3% holding the top rank of 1, reflecting their prominence in Amazon's search results.
However, the data also showed that a large share of recommended products (75th percentile and above) had an organic rank of 999, meaning they were not detectable by AMALYTIX. This suggests that Rufus recommendations do not exclusively rely on a product’s visibility in Amazon’s traditional search.
The presence of both high-ranking and undetectable products highlights the complexity of how Rufus selects recommendations, emphasizing the need for brands to optimize multiple aspects of their listings beyond organic rank.
11. Fulfillment Type

- FBA (Fulfilled by Amazon): 94.2%
- FBM (Fulfilled by Merchant): 5.8%
FBA was overwhelmingly dominant among recommended products, accounting for over 94% of listings. This indicates a strong preference for FBA listings in Rufus’s recommendations, likely due to the benefits associated with Amazon's fulfillment network, such as faster shipping times and Prime eligibility.
Among the FBM products, 93.5% were not Prime eligible, and notably, not a single seller-fulfilled Prime product was recommended. This highlights that Prime eligibility plays a significant role in the recommendation system, and the absence of seller-fulfilled Prime listings suggests a potential limitation in their or visibility.
Additionally, a comparison of the recommended FBM product listings with the entire dataset showed no major deviations in other listing attributes.
12. Prime Eligibility
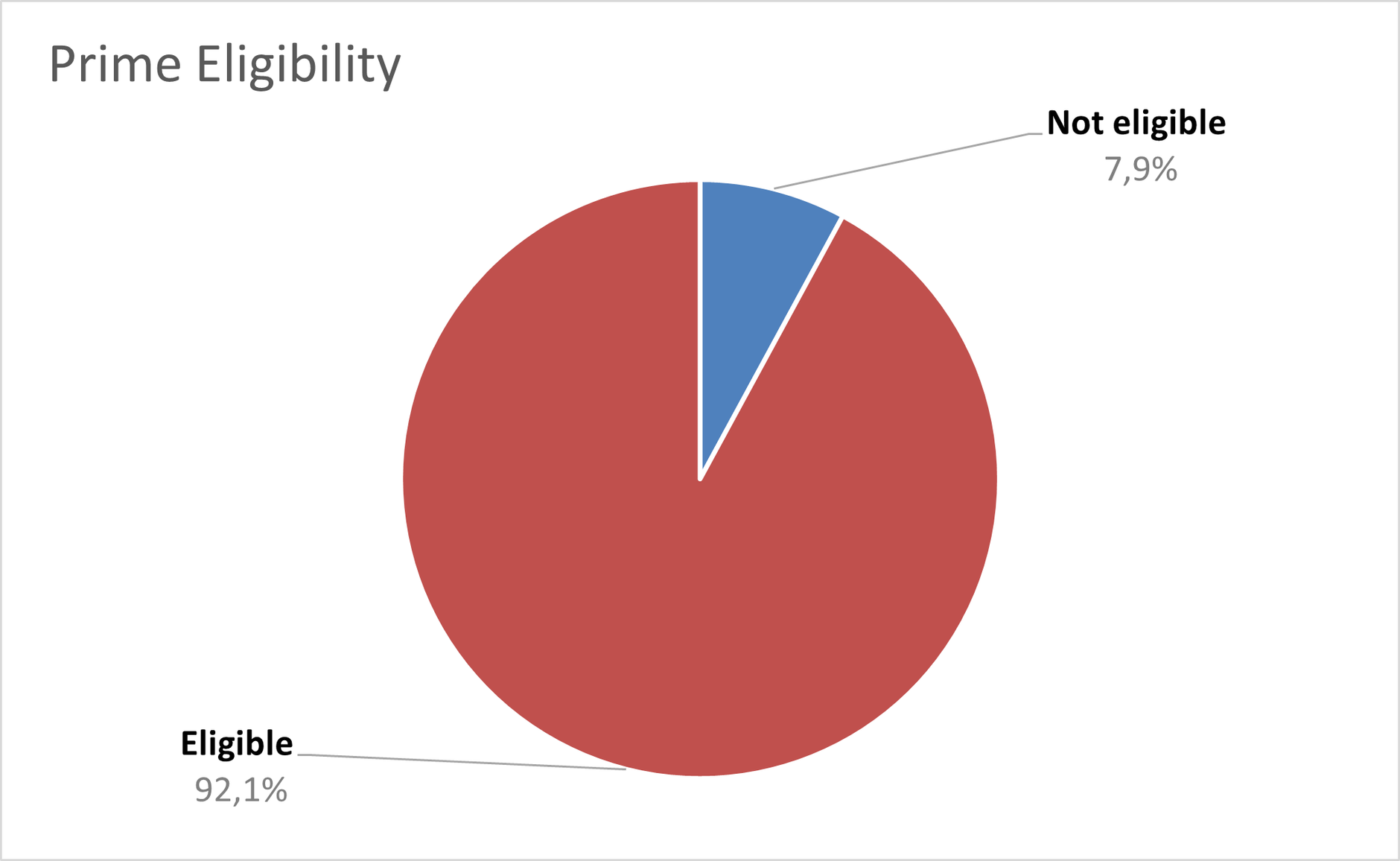
- Prime Eligible Products: 92.1%
- Non-Prime Products: 7.9%
Consequently, the overwhelming majority of recommended products (92.1%) were Prime eligible, emphasizing the importance of offering fast and reliable shipping options to customers. Non-Prime products accounted for only a small fraction (7.9%) of recommendations, suggesting that Prime eligibility significantly enhances a product's likelihood of being featured by Rufus.
Sellers should prioritize enrolling their products in the Prime program to improve their visibility in recommendations and appeal to Amazon’s loyal Prime customer base.
13. Product Availability Status
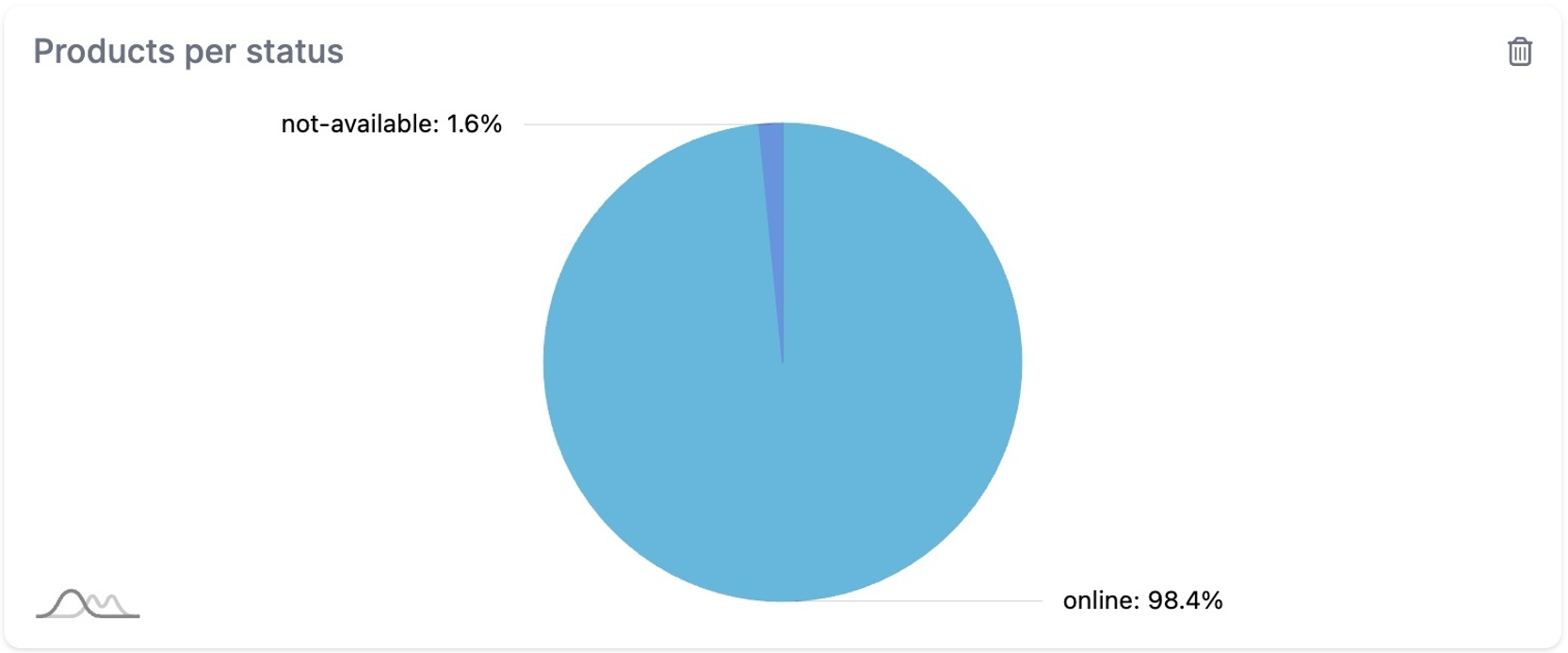
- Online (Available): 98.4%
- Not Available: 1.6%
Nearly all recommended products were listed as "online," indicating that Rufus predominantly selects products that are readily available for purchase. Only a very small fraction of recommended listings were marked as "not-available," suggesting that product availability is a critical factor in determining recommendations.
For brands, ensuring consistent product availability is crucial for maintaining visibility in Rufus’s recommendations.
14. Amazon’s Role in Recommended Listings
To analyze Amazon's involvement in recommended products, we examined two indicators: whether Amazon has an offer on the product and whether Amazon holds the Buy Box.
Indicator 1: Amazon Has an Offer
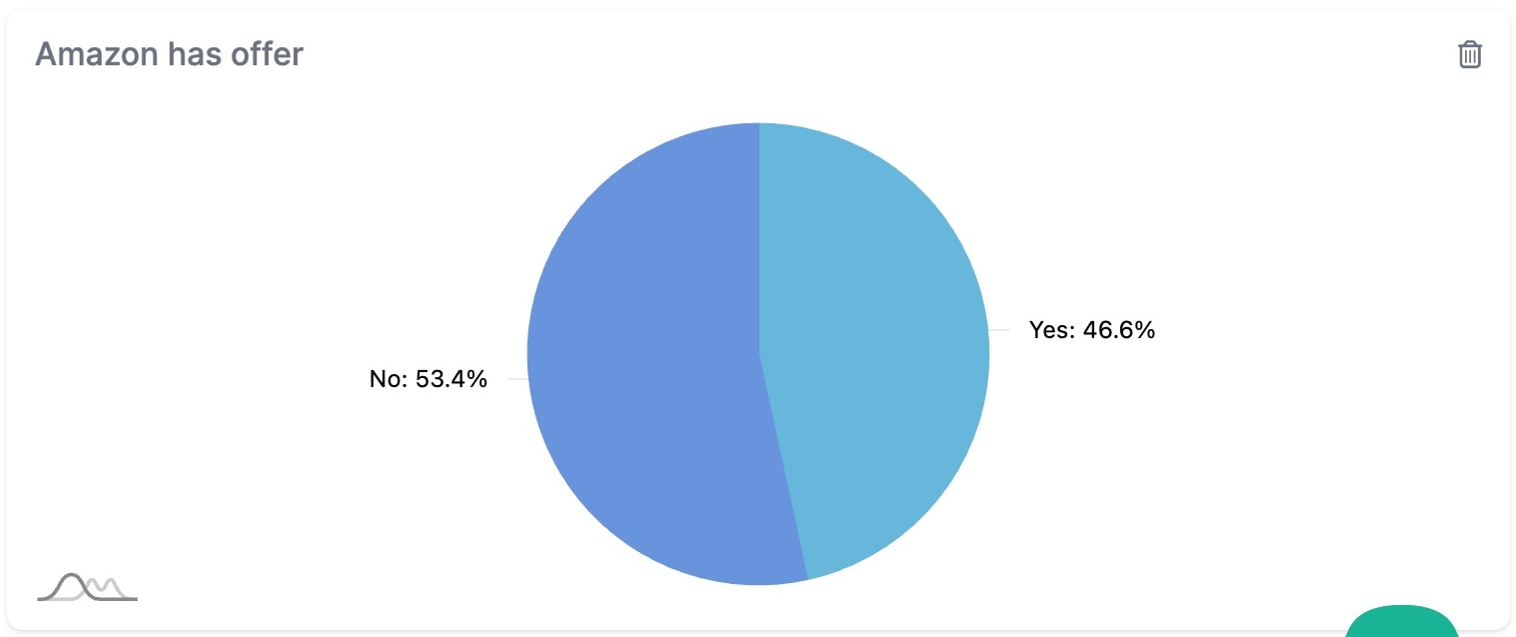
- Products sold by Amazon: 46.6%
- Products sold by 3P sellers: 53.4%
Indicator 2: Amazon Has the Buy Box
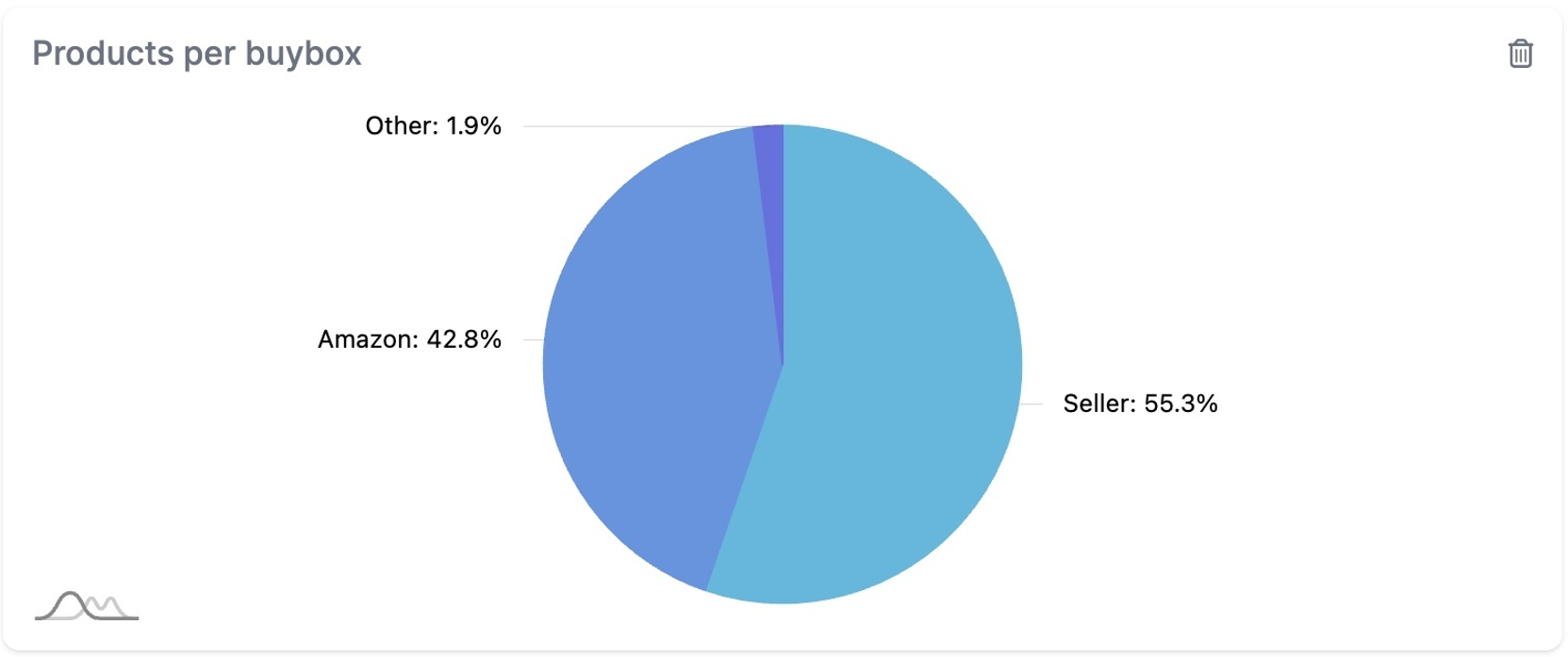
- Products with Amazon in the Buy Box: 42.8%
- Products with 3P sellers in the Buy Box: 55.3%
- Other: 1.9%
Surprisingly, the majority of recommended products were not sold by Amazon. Over 53% of recommendations were for products sold by 3P sellers, and in 55% of cases, 3P sellers held the Buy Box. This indicates that Rufus’s recommendations are not overly skewed toward Amazon listings, highlighting significant opportunities for 3P sellers to appear prominently in recommendations.
15. Consistency and Reproducibility
Our analysis, based on running repeated queries for some of the identified keywords, reveals that Amazon Rufus demonstrates a high degree of consistency in its recommendations. Across five test runs per keyword, certain products were consistently recommended in every iteration. On average, 2–3 ASINs per keyword appeared in 100% of the test runs, showing Rufus’s clear preference for specific listings that align with its ranking criteria.
However, Rufus also introduces variability in its results, with additional ASINs appearing less frequently. For most keywords, 4–6 ASINs were recommended inconsistently, showing up in fewer than all five runs. This suggests that Rufus balances consistent recommendations of highly relevant products with a degree of diversity to broaden the user’s options.
The reproducibility of Rufus’s results highlights its reliability in promoting specific products while maintaining some flexibility. For sellers, this means that optimizing key listing attributes can lead to consistent recommendations, while occasional variability allows opportunities for other products to be included as well.
Discover AMALYTIX
Curious how we conducted this analysis? With AMALYTIX, you gain powerful insights into your product listings, including advanced content monitoring, performance tracking, and data visualization tailored to Amazon. See how AMALYTIX can help optimize your Amazon strategy—start your free 14-day trial today!
Conclusion
Our analysis of Amazon Rufus’s recommendations provides a detailed look at the attributes that appear to influence its AI-driven product selections.
Key findings include the importance of utilizing nearly the maximum allowable title length, a robust image count of 6–9, and leveraging enhanced content such as A+ or A+ Premium descriptions. While high product ratings and substantial review counts were common among recommended products, Rufus also demonstrated a willingness to recommend listings with minimal reviews if other aspects were strong. Prime eligibility and FBA fulfillment emerged as dominant factors, underscoring the value of fast shipping and reliable service in the recommendation process.
Interestingly, Rufus’s recommendations showed significant diversity across brands, suggesting that its algorithm prioritizes variety over brand dominance. However, badges like Amazon’s Choice, which appeared on nearly half of the recommended products, clearly play a significant role in enhancing product visibility.
Overall, this analysis highlights the nuanced and multifaceted nature of Rufus’s recommendations, offering sellers and vendors valuable insights into optimizing their product listings. While correlation does not imply causation, understanding these trends can guide strategic decisions to better align listings with Rufus’s AI-driven logic.
As Amazon Rufus continues to evolve, ongoing analysis will be crucial for adapting to its recommendation patterns and leveraging its potential to improve product visibility.